Department of Translational Surgical Oncology
We are an interdisciplinary research group including disciplines like computer science, engineering and medicine. Our research goal is to develop and evaluate computer- and robotic-assisted systems which optimize the surgical therapy of the individual patient by turning the available data into useful information.
Therefore, we are investigating the entire process chain along the surgical treatment path including pre-, intra- and postoperative patient information. Eligibility and success rates of surgeries can be improved using personalized digital representations of the patient and enable the deployment of this data in the operating room (OR), e.g. by visualization of planning data or the prediction of complications.
The research and methodological focuses are surgical workflow analysis, soft-tissue navigation and intraoperative visualization as well as surgical training and surgical data science. These methods allow for the first time a context-aware assistance in the OR of the future, which acts as an automatic information filter, avoids information overflow, adapts to the current needs of the surgeon and therefore provides the right information at the right time. In order to achieve this goal the close collaboration with interdisciplinary partners, in particular physicians, is necessary. Overall such systems have the potential to improve patient outcome and open up new possibilities to operate.
Contact
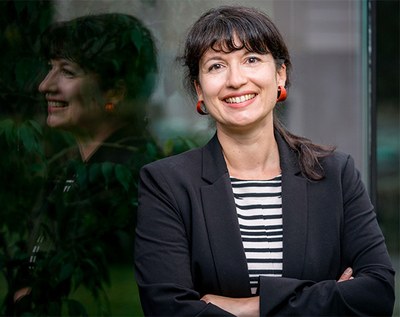
Prof. Dr. Stefanie Speidel
Translational Surgical Oncology
Phone: +49 (0)351 458 5413
E-Mail: Stefanie.speidel(at)nct-dresden.de
- Rivoir, D., Funke, I., & Speidel, S. (2024). On the pitfalls of batch normalization for end-to-end video learning: A study on surgical workflow analysis. Medical Image Analysis, 103126.
- Kolbinger, F. R., Rinner, F. M., Jenke, A. C., Carstens, M., Krell, S., Leger, S., ..., Speidel, S., & Bodenstedt, S. (2023). Anatomy segmentation in laparoscopic surgery: comparison of machine learning and human expertise–an experimental study. International Journal of Surgery, 109(10), 2962-2974.
- Wagner, M., Müller-Stich, B. P., Kisilenko, A., Tran, D., Heger, P., Mündermann, L., ... & Speidel, S., Bodenstedt, S. (2023). Comparative validation of machine learning algorithms for surgical workflow and skill analysis with the heichole benchmark. Medical image analysis, 86, 102770.
- Bhasker, N., Kolbinger, F. R., Skorobohach, N., Zwanenburg, A., Löck, S., Weitz, J., ... & Kühn, J. P. (2023). Prediction of clinically relevant postoperative pancreatic fistula using radiomic features and preoperative data. Scientific Reports, 13(1), 7506.
- Kolbinger, F. R., Bodenstedt, S., Carstens, M., Leger, S., Krell, S., Rinner, F. M., ... & Speidel, S. (2023). Artificial Intelligence for context-aware surgical guidance in complex robot-assisted oncological procedures: An exploratory feasibility study. European Journal of Surgical Oncology, 106996.